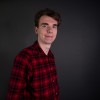
Filip Milic
DATA ENGINEER
Elaborate data processing pipelines are relied upon to work like clockwork. But much like clocks, they always have a tendency to break.
The importance and sheer volume of data being produced on a daily basis is nothing short of incredible. We are at a point where we can tell an entire person’s story through it – their likes, their dislikes, the people they interact with, the things they want, the things they don’t even KNOW they want – and that’s just one person. When we scale it up to billions, the fact that data-exchange flows as well as it does is nothing short of an engineering miracle.
There is always a but…
Of course, what separates an engineering miracle from an actual miracle is its imperfections. And it has quite a few. Elaborate data processing pipelines are relied upon to work like clockwork. But much like clocks, they always have a tendency to break. Be it miscommunication between teams, badly formatted data, unpredictable server failures or just good old-fashioned bugs, the fact of the matter is that, in a field like data engineering, something going wrong is almost a given.
And as said pipelines slowly migrate to platforms that are evolving faster than them, our priorities should shift from standard prevention of failures, to mechanisms allowing swift and accurate recovery from them.
Persistor is just such a tool.
Tackling the root cause
We have worked as a consultancy firm for several years now, working for many different clients. In that time, we came to realize there were a lot of overlapping – if not downright identical – issues between all of them. And instead of giving the same advice and rebuilding the same tools over and over again, we decided to strike the issues at their core.
With Persistor in particular, it was loss of messages – raw data – due to some part of the pipeline failing. Messages that, for one reason or another, could not simply be re-sent, meaning the loss was permanent. And given the aforementioned importance and volume of data, even a “minor” loss amount-wise could turn out to be a major one in the grand scheme of things. And that was unacceptable.
Sometimes it just better to K.I.S.S.
The ultimate solution to the problem is really as simple as the Persistor itself. If we don’t want to lose messages – we’ll back them up somewhere as they arrive to the pipeline!
All the Persistor does, at its core, is store the raw message payloads to storage blobs as they arrive through your cloud messaging services. It’s cheap, it’s fast, it’s efficient and it’s modular. The last part being of particular importance to us: you should think of Persistor as an addition to already existing pipelines. It works completely independently and does not disrupt whatever workflows are already running.
It does not alter your data: Persistor reads. Persistor writes. That’s all there is to it.
For data’s sake…
While the obvious benefit is that it makes for an efficient fail-safe, the practice of storing raw data has undeniable benefits to begin with. Data analysis and data science rely on extracting and processing certain parts of the data, tossing the other ones aside as redundant. However, years or maybe even as soon as months down the line, as your business and clientele expand, you may come to realize that redundant data may not have been so redundant after all. And if you don’t happen to have the original data – well. It’s unfortunate, to say the least.
That’s not even mentioning the benefit of smoother communication between clients and developers. As a developer myself, I know that I can understand and act much faster on a client’s demands when I have a firmer grasp of what is and isn’t possible to do. And seeing the actual data is the most important part of that! Not to mention, clients themselves can be recommended how the information gathering could be expanded in useful ways.
Maybe we’re stating the obvious here, but it’s important to understand that a lot of this stuff is still in its infancy, and a lot of the more “obvious” things still haven’t been recognized and set in stone. But we’re hoping that, with Persistor, at least one of those things now will be.
It should also be noted that Persistor itself is highly-scalable, due to the fact that it’s built as a serverless component. We designed it specifically around Cloud functions for GCP and Azure functions so the provided service can take full advantage of their scaling mechanisms. And as we slowly work towards a standalone docker component, users themselves will be able to define and control the level of scalability, depending on their needs and systems.
Finally – and perhaps most importantly – Persistor is an open-source project.
Sharing is caring
Persistor is just the first step in a much larger goal of advancing our community of data engineers. And, in turn, we hope that they’ll reach back and help us improve wherever possible. That is why the open-source approach was so important to us.
Of course, one of the most common misconceptions about open-source is that the code is free until we decide it suddenly isn’t. We want to assure everyone that is not the case – we are using the Apache license that guarantees you can use the Persistor from now, until the end of time, without paying us a dime. This is a tool built for you. Stopping or disincentivizing you from using it defeats the point.
The Persistor is designed for GCP and Azure messaging services. Both variants available on our GITHUB!